Integrating cross-scale analysis in the spatial and temporal domains for classification of behavioral movement
Keywords:
cross-scale movement analysis, spatial scaling, temporal scaling, movement parameters, machine learning, zebrafish swimming, behavioral pharmacology, drug screeningAbstract
Since various behavioral movement patterns are likely to be valid within different, unique ranges of spatial and temporal scales (e.g., instantaneous, diurnal, or seasonal) with the corresponding spatial extents, a cross-scale approach is needed for accurate classification of behaviors expressed in movement. Here, we introduce a methodology for the characterization and classification of behavioral movement data that relies on computing and analyzing movement features jointly in both the spatial and temporal domains. The proposed methodology consists of three stages. In the first stage, focusing on the spatial domain, the underlying movement space is partitioned into several zonings that correspond to different spatial scales, and features related to movement are computed for each partitioning level. In the second stage, concentrating on the temporal domain, several movement parameters are computed from trajectories across a series of temporal windows of increasing sizes, yielding another set of input features for the classification. For both the spatial and the temporal domains, the ``reliable scale'' is determined by an automated procedure. This is the scale at which the best classification accuracy is achieved, using only spatial or temporal input features, respectively. The third stage takes the measures from the spatial and temporal domains of movement, computed at the corresponding reliable scales, as input features for behavioral classification. With a feature selection procedure, the most relevant features contributing to known behavioral states are extracted and used to learn a classification model. The potential of the proposed approach is demonstrated on a dataset of adult zebrafish (Danio rerio) swimming movements in testing tanks, following exposure to different drug treatments. Our results show that behavioral classification accuracy greatly increases when firstly cross-scale analysis is used to determine the best analysis scale, and secondly input features from both the spatial and the temporal domains of movement are combined. These results may have several important practical applications, including drug screening for biomedical research.
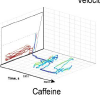
Downloads
Published
Issue
Section
License
Copyright (c) 2014 Ali Soleymani, Jonathan Cachat, Kyle Robinson, Somayeh Dodge, Allan Kalueff, Robert Weibel

This work is licensed under a Creative Commons Attribution 4.0 International License.
Articles in JOSIS are licensed under a Creative Commons Attribution 3.0 License.