Data-driven agriculture for rural smallholdings
Keywords:
agriculture, survey, semantics, data science, economicsAbstract
Spatial information science has a critical role to play in meeting the major challenges facing society in the coming decades, including feeding a population of 10 billion by 2050, addressing environmental degradation, and acting on climate change. Agriculture and agri-food value-chains, dependent on spatial information, are also central. Due to agriculture's dual role as not only a producer of food, fibre and fuel, but also as a major land, water and energy consumer, agriculture is at the centre of both the food-water-energy-environment nexus and resource security debates.
The recent confluence of a number of advances in data analytics, cloud computing, remote sensing, computer vision, robotic and drone platforms, and IoT sensors and networks have lead to a significant reduction in the cost of acquiring and processing data for decision support in the agricultural sector. When combined with cost-effective automation through development of swarm farming technologies, the technology has the potential to decouple productivity and cost efficiency from economies of size, reducing the need to increase farm size to remain economically viable.
We argue that these pressures and opportunities are driving agricultural value-chains towards high-resolution data-driven decision-making, where even decisions made by small rural landowners can be data-driven. We survey recent innovations in data, especially focusing on sensor, spatial and data mining technologies with a view to their agricultural application; discuss economic feasibility for small farmers; and identify some technical challenges that need to be solved to reap the benefits. Flexibly composable information resources, coupled with sophisticated data sharing technologies, and machine learning with transparently embedded spatial and aspatial methods are all required.
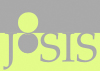
Downloads
Published
Issue
Section
License
Copyright (c) 2020 Kerry Taylor, Martin Amidy

This work is licensed under a Creative Commons Attribution 4.0 International License.
Articles in JOSIS are licensed under a Creative Commons Attribution 3.0 License.